by Bochen Guan, Saide Wu and Cheng Xiang
STATISTICAL ANALYSIS
Statistical analysis was performed using MATLAB (version 2017a, MathWorks, Natick, MA) and MedCalc (version 14.8; MedCalc Software, Ostend, Belgium) with statistically significance defined as a p-value less than 0.05. Contingency tables and sensitivity and specificity for the musculoskeletal radiology fellows and cartilage lesion detection system for determining ACL tear on each image patch were calculated using the interpretation of the musculoskeletal radiologist as the reference standard. Receiver operating characteristic (ROC) analysis was used to further evaluate the diagnostic performance of the ACL tear detection system. For the ROC analysis, the area under the curve (AUC) was calculated for evaluation of the ACL tear diagnosis system with the AUCs using a nonparametric approach. Â The Youden index was used to determine optimal sensitivity and specificity.
EVALUATION OF CLINICALÂ RADIOLOGISTS
To compare the diagnostic performance of the ACL diagnosis system with clinical radiologists, a musculoskeletal radiology fellow who completed residency and had two months of fellowship experience and an experienced musculoskeletal radiologist working in UW health for more than three years independently reviewed the sagittal fat-suppressed T2-weighted fast spin-echo and sagittal intermediate-weighted fast spin-echo images of all 100 testing patients side-by-side using the same customized software as for training reference. The musculoskeletal radiology fellow and the experienced musculoskeletal radiologist used all two sequences together to determine the ACL tear of a subject.
For the fellow and radiologist, the sensitivity (95%CI) was 94.44% (81.3% to 99.3%) and 97.22% (85.5% to 99.9%) respectively, while the specificity (95%CI) was 98.44% (91.6% to 100%) and 98.44% (91.6% to 100.0%) respectively. In comparison, the optimal threshold by the Youden index for sensitivity (95%CI) and specificity (95%CI) for our system was 88.89% (73.9% - 96.9%) and 98.44% (91.6% - 100.0%) respectively for the IW-FSE images and the T2-FSE images. The AUC (95%CI) of our system was 0.942 (0.876 to 0.979, p< 0.0001) for the the sagittal fat-suppressed T2-weighted fast spin-echo and sagittal intermediate-weighted fast spin-echo images (Figure).
Figure X: ROC curve illustrating the diagnostic performance of the DL method for detecting surgically confirmed ACL tears using sagittal IW-FSE images and T2-FSE images. The solid line is the ROC curve and dotted lines are the 95% confidence bounds. The AUC value of 0.942 is significant larger than 0.5 (p<0.0001). Â The diagnostic performance of the DL method is compared to a musculoskeletal radiology fellow (blue circle) and experienced musculoskeletal radiologist (red triangle).
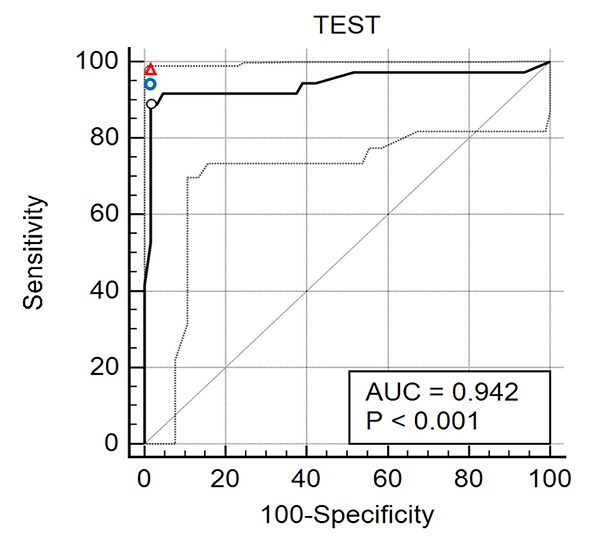
Figure 5 ROC curve illustrating the diagnostic performance of the DL method for detecting surgically confirmed ACL tears using sagittal IW-FSE images and T2-FSE images. The solid line is the ROC curve and dotted lines are the 95% confidence bounds. The AUC value of 0.942 is significant larger than 0.5 (p<0.0001). The diagnostic performance of the DL method is compared to a musculoskeletal radiology fellow (blue circle) and experienced musculoskeletal radiologist (red triangle).
COMPARISON WITH OTHER DEEP LEARNING APPROACHES
If we consider ACL tear detection as an ordinary object detection problem, several related detection/classification deep learning methods might work for this problem. We implement two common deep learning object detection approaches to detect ACL tear for the same 100 testing subjects and compare the testing results with our model. A multi-class mask can be created for each single slice with the following values for the other deep learning methods: 0=background, 1=with ACL tear, 2=without ACL tear. The output ACL tear probability of each subject is computed by
is the number of slides detected with ACL tear; Â represents the total number of slides in a subject; Â is the number of slides detected with background. Thus, Â is the number of slides be detected with ACL (might existing ACL tear or not).
Table shows the results of applying two common object detection algorithms for this problem. The optimal threshold by the Youden index for sensitivity (95%CI) and specificity (95%CI) for the other deep learning methods are not acceptable for a clinical diagnosis. However, our model shows a better performance and gives higher testing accuracy for the 100 subjects experiment.

Table 1. Testing results of our model and two common deep learning methods.